How AI Database Systems Are Revolutionizing Solar Backup Maintenance
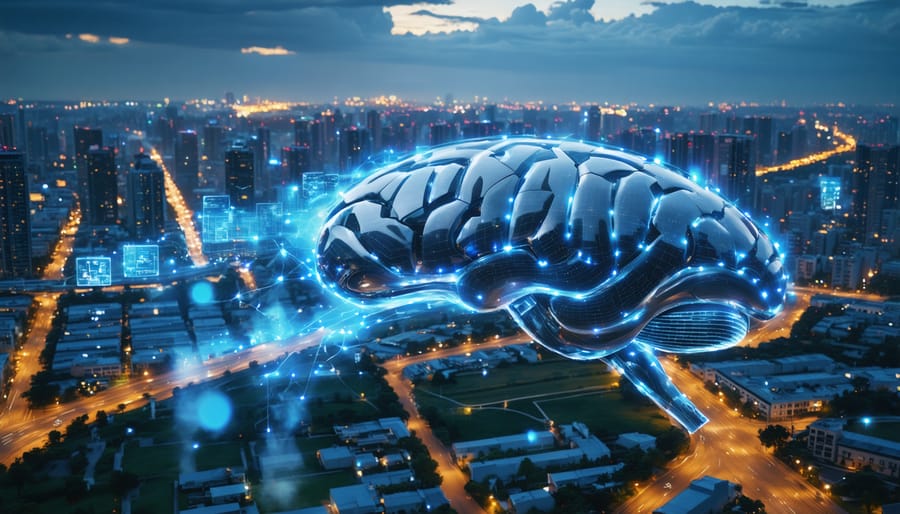
Artificial Intelligence is revolutionizing database management systems, ushering in a new era of intelligent data handling that transcends traditional capabilities. Modern AI-powered database systems autonomously optimize queries, predict maintenance needs, and adapt to changing workloads in real-time – transforming how organizations manage and leverage their data assets. These systems combine machine learning algorithms with advanced analytics to deliver unprecedented levels of automation, security, and performance optimization.
For technical decision-makers in Europe’s expanding digital infrastructure, AI database management systems offer a compelling solution to handle exponentially growing data volumes while reducing operational complexity. By incorporating predictive maintenance, automated tuning, and intelligent resource allocation, these systems are particularly valuable for mission-critical operations in sectors ranging from renewable energy to industrial manufacturing.
As organizations navigate the transition toward smarter data management solutions, AI-driven systems represent not just an upgrade to existing infrastructure, but a fundamental shift in how we approach database administration and optimization.
The Evolution of Database Management in Solar Energy
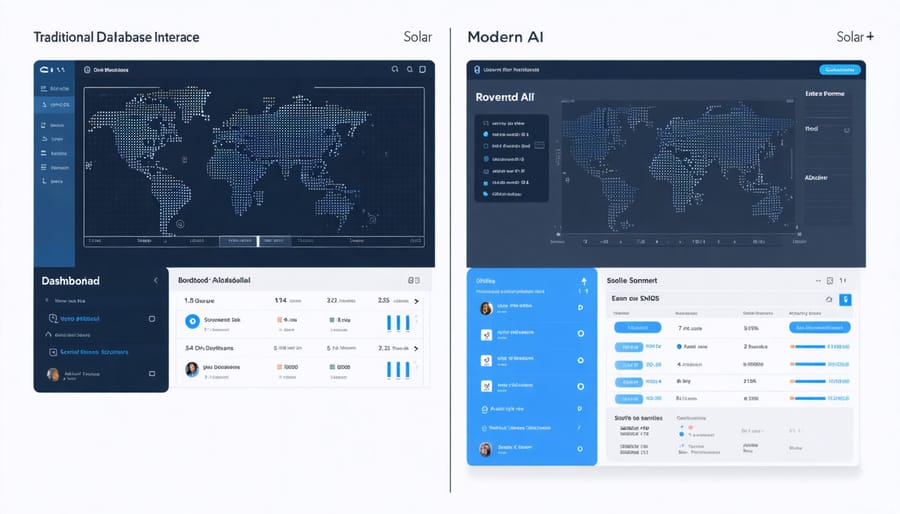
Traditional Database Challenges
Traditional database management systems face several critical challenges in maintaining solar backup systems effectively. Regular manual updates, data consistency issues, and complex query processing often lead to operational inefficiencies. System administrators frequently struggle with time-consuming backup schedules, resource-intensive maintenance routines, and the risk of human error during critical operations. Storage capacity management becomes increasingly complex as data volumes grow, while performance optimization requires constant attention and adjustment. The challenge of maintaining data integrity across multiple systems and ensuring seamless disaster recovery capabilities adds another layer of complexity. These limitations can significantly impact the reliability and efficiency of solar energy storage solutions, particularly during peak demand periods or system emergencies.
AI-Driven Solutions
AI-powered database management systems are revolutionising how organisations handle complex data operations in the solar energy sector. These intelligent systems leverage machine learning algorithms to automate routine tasks, predict maintenance needs, and optimise data storage patterns. By analysing historical data patterns, AI databases can automatically adjust storage configurations, implement smart indexing, and optimise query performance without human intervention.
One of the key advantages is the system’s ability to self-tune and adapt to changing workloads. For instance, when managing solar installation data across multiple European facilities, AI databases can automatically distribute resources based on peak usage times and seasonal variations. This intelligent resource allocation ensures optimal performance while reducing operational costs.
The technology also excels in predictive maintenance and error prevention. By continuously monitoring database health metrics, AI systems can identify potential issues before they impact operations. This proactive approach is particularly valuable for solar energy facilities that rely on real-time data processing for performance monitoring and energy distribution management.
Furthermore, AI databases incorporate advanced security features that evolve with emerging threats, making them significantly more resilient than traditional systems. This enhanced security framework is crucial for protecting sensitive solar infrastructure data and maintaining compliance with European data protection regulations.
Core Components of AI Database Management
Real-time Data Processing
AI-powered database management systems excel at processing real-time data streams from solar backup systems, ensuring continuous monitoring and optimal performance. These systems employ sophisticated algorithms to analyse incoming data packets instantly, identifying patterns and anomalies that might affect system efficiency.
The real-time processing capability allows for immediate response to critical events, such as sudden voltage fluctuations or unexpected system behaviour. By implementing parallel processing techniques, AI systems can simultaneously handle multiple data streams from various backup components while maintaining processing speed and accuracy.
Modern AI database systems utilise edge computing architecture to reduce latency and enable faster decision-making. This approach processes data closer to its source, ensuring minimal delay between data collection and analysis. The system continuously updates its knowledge base, learning from new data patterns to improve its predictive capabilities and operational efficiency.
For solar energy installations, this translates to enhanced backup reliability through instantaneous monitoring of battery health, charge cycles, and power distribution. The AI system maintains detailed logs of all processing activities, creating a comprehensive audit trail for compliance and maintenance purposes while optimising storage resources through intelligent data compression and archival strategies.
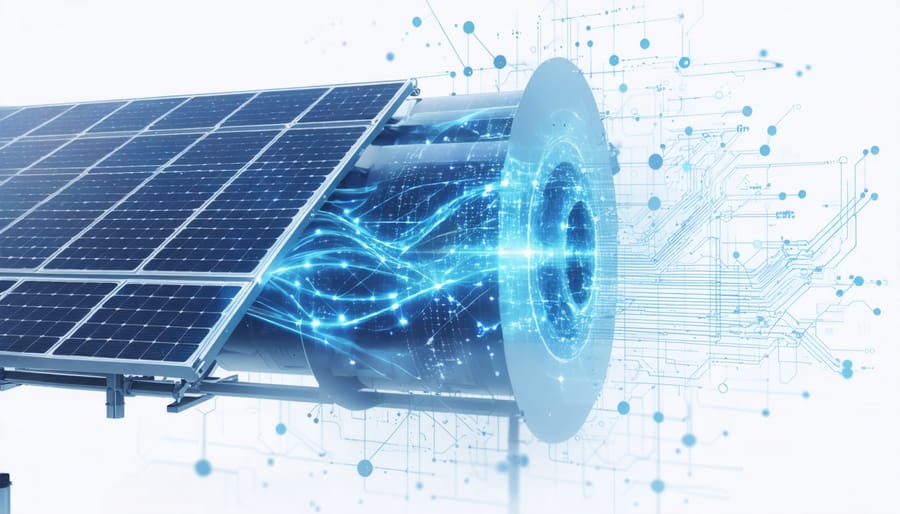
Pattern Recognition Algorithms
Pattern recognition algorithms in AI database management systems serve as intelligent monitors that continuously analyze system performance and data patterns. These sophisticated algorithms employ machine learning techniques to identify potential anomalies, predict maintenance needs, and optimize database operations in real-time.
The core functionality relies on three primary recognition methods: statistical pattern analysis, which examines historical data trends; behavioral recognition, which monitors current system activities; and predictive modeling, which forecasts potential issues before they impact performance.
These algorithms are particularly valuable in solar energy applications, where they can detect subtle changes in energy storage patterns, identify potential equipment malfunctions, and optimize database performance based on usage patterns. For instance, they can recognize when certain query patterns might lead to system bottlenecks or when storage allocation needs adjustment based on seasonal solar production variations.
By implementing these recognition systems, facility managers can ensure more efficient database operations while maintaining optimal performance of their solar energy infrastructure. The algorithms continuously learn and adapt, improving their accuracy over time and providing increasingly refined insights for system optimization.
Predictive Analytics Integration
Modern AI database management systems leverage historical performance data to create sophisticated predictive models that prevents costly system failures through proactive maintenance scheduling. By analyzing patterns in equipment performance, energy output fluctuations, and component degradation rates, these systems can accurately forecast when maintenance will be needed. The AI continuously learns from new data inputs, refining its predictions and becoming more accurate over time. This approach enables facility managers to optimize maintenance schedules, reduce unexpected downtime, and extend the lifespan of solar backup systems. The predictive capabilities also help in budget planning by anticipating resource requirements and scheduling maintenance during optimal weather conditions.
Implementation Benefits for Solar Systems
Enhanced System Reliability
AI-powered database management systems significantly enhance the reliability of solar energy installations by implementing intelligent backup protocols and predictive maintenance strategies. Through advanced machine learning algorithms, these systems continuously monitor system performance, automatically detecting and responding to potential issues before they impact operations. This proactive approach is crucial for building resilient energy systems that can maintain consistent performance even under challenging conditions.
The AI systems employ sophisticated data analysis to optimize backup schedules, ensuring critical information is protected while minimizing system downtime. By learning from historical performance patterns, these intelligent systems can predict peak usage periods and automatically adjust backup processes to occur during low-demand intervals. This optimization not only improves system efficiency but also reduces the risk of data loss or system failures.
Furthermore, AI-driven monitoring provides real-time alerts and automated recovery procedures, enabling quick response to potential system irregularities. This enhanced reliability translates into improved uptime statistics and more stable operations for solar energy facilities across Europe, ultimately contributing to a more dependable renewable energy infrastructure.
Cost Optimization
AI-driven predictive maintenance in database management systems significantly reduces operational expenses by preventing costly system failures and optimizing resource allocation. Through advanced analytics and machine learning algorithms, these systems can accurately forecast maintenance needs, helping facilities minimize downtime and extend equipment lifespan. This proactive approach has shown to reduce maintenance costs by up to 30% while improving system reliability.
For solar energy installations, AI database management systems help optimize solar battery backup costs through intelligent monitoring and predictive maintenance scheduling. The system analyzes performance patterns, weather data, and usage trends to recommend optimal maintenance timing, preventing unnecessary interventions while ensuring critical issues are addressed before they escalate.
Additionally, these systems automate resource allocation, reducing labor costs and minimizing human error in maintenance scheduling. The resulting efficiency gains and reduced maintenance requirements typically deliver a return on investment within 12-18 months of implementation.
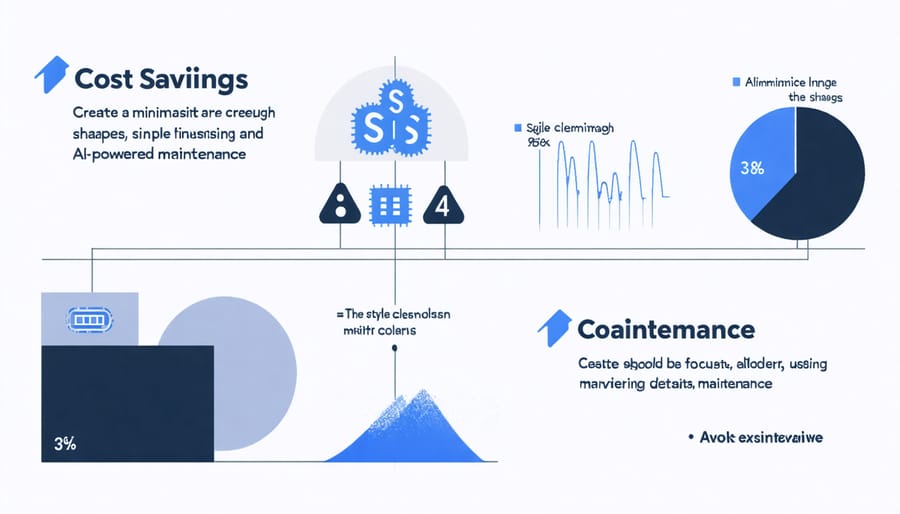
Sustainability Impact
AI-powered database management systems significantly reduce the environmental footprint of data centers through intelligent resource allocation and optimized maintenance schedules. By predicting maintenance needs with precision, these systems minimize unnecessary servicing, reducing both energy consumption and waste from replacement parts. The predictive capabilities ensure equipment operates at peak efficiency, leading to an estimated 15-25% reduction in energy usage across European data facilities.
The implementation of AI-driven maintenance schedules has shown remarkable sustainability benefits, including decreased carbon emissions from reduced maintenance-related travel and extended equipment lifespan through proactive care. Studies from leading European research institutions indicate that smart database management systems can decrease electronic waste by up to 30% through better hardware utilization and timely interventions.
Furthermore, these systems contribute to sustainability goals by optimizing cooling systems, managing power distribution, and automating resource-intensive tasks during off-peak hours, creating a more environmentally responsible approach to data management.
Future Prospects and Integration
The integration of AI database management systems with emerging technologies continues to reshape the solar energy landscape. Machine learning algorithms are becoming increasingly sophisticated, enabling predictive maintenance capabilities that can anticipate system failures before they occur. This advancement is particularly relevant for European solar installations, where weather patterns and regulatory requirements demand precise monitoring and management.
Smart grid integration represents another promising frontier, with AI database systems facilitating seamless communication between solar installations and power distribution networks. These systems are evolving to handle real-time data processing at unprecedented scales, supporting the growing complexity of renewable energy networks across Europe.
Edge computing integration is gaining momentum, allowing for faster data processing closer to the source. This development is especially valuable for remote solar installations, reducing latency and enabling more responsive system management. Additionally, blockchain technology is being incorporated into AI database systems, enhancing data security and enabling transparent energy trading platforms.
Looking forward, we can expect to see increased automation in database management, with AI systems becoming more autonomous in decision-making processes. The integration of natural language processing will make these systems more accessible to non-technical users, while quantum computing developments promise to revolutionize data processing capabilities.
These advancements are laying the groundwork for more efficient, reliable, and sustainable solar energy solutions, supporting Europe’s transition to renewable energy sources.
The integration of AI database management systems in solar backup maintenance represents a significant leap forward for European energy infrastructure. By automating monitoring processes, predicting maintenance needs, and optimising system performance, these intelligent solutions are revolutionising how we maintain and manage solar backup systems. The enhanced efficiency and reduced operational costs directly benefit homeowners and businesses alike, while contributing to the broader goals of energy sustainability across Europe. As we continue to embrace renewable energy solutions, the role of AI in database management becomes increasingly crucial for ensuring reliable backup power systems. The future of solar maintenance lies in these smart, adaptive systems that not only streamline operations but also provide comprehensive insights for better decision-making. This technological advancement marks a new era in sustainable energy management, promising more reliable, efficient, and cost-effective solar backup solutions for European communities.
Leave a Reply